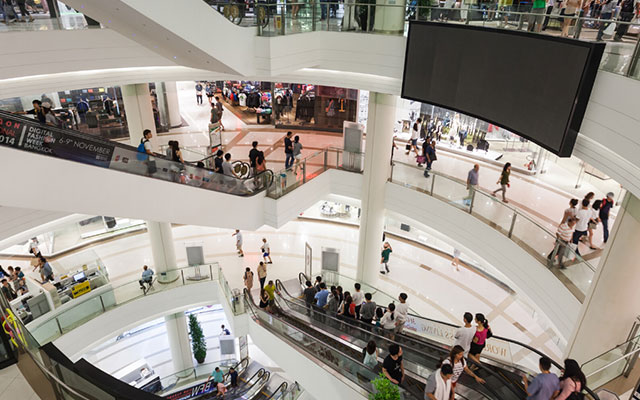
Leveraging Computer Vision Techniques for Crowd Analysis in 2020
Crowd analysis as one of the core computer vision applications is based on the analysis and interpretation of data obtained from studying the movement of a crowd or a group. It is about collecting and aggregating insightful information about crowd mobility in places of mass gathering from airports to metro areas, train stations, etc.
Crowd analysis has been generating a lot of buzz in recent times. This is all thanks to its diverse applications for video surveillance. And while anyone could be led to believe that the straightforward approach and techniques used for non-crowded scenes could also be applied to crowds, there are far too many challenges to make this generate significant results. It is for this reason that crowd analysis has emerged over time as a central topic in computer vision research for crowd surveillance. In this guide, we take a brief look at crowd analysis and its applications.
Crowd analysis and its application
Crowd analysis as one of the core computer vision applications is based on the analysis and interpretation of data obtained from studying the movement of a crowd or a group. It is about collecting and aggregating insightful information about crowd mobility in places of mass gathering from airports to metro areas, train stations, etc.
This requires a series of computer vision applications and techniques from people tracking to crowd density estimation, event detection, as well as simulation and validation. Common instances of crowd analysis and its application include;
- Crowd management: as in the analysis of crowd scenes in mass gatherings to ensure mobility, develop crowd management strategies, and ensure public safety.
- Public space design: as in the application of crowd analytics to understand crowd dynamics and provide valuable input into the design of public spaces such as train stations, theaters, airport terminals, and more, for improved efficiency and safety at mass gatherings.
- Virtual environment: as in the validation, application, and performance of mathematical models used in crowd simulations.
- Intelligent environment: as in making well-informed choices on how to manage a crowd in a public space based on data from crowd behavior.
Crowd analysis: how does computer vision work?
While crowd analysis and its applications are quite diverse, this practice involves a three-pronged approach that includes pre-processing, object tracking, as well as event/behavior recognition.
- The pre-processing method is comprised of four other strategies that include; a pixel-based analysis that leverages local features to estimate crowd population; a texture-based analysis that analyses high-level features to estimate the number of people in a scene rather than identifying individuals; an object-level analysis that analysis individual objects and boasts a level of accuracy that’s significantly higher than both pixel-based and texture-based analysis; and a frame-level analysis that simulates the behavior of a crowd scene with the field of view.
- Object tracking, on the other hand, involves crowd analysis strategies like region-based, active contour, feature-based, and model-based approaches that aim to minimize some of the constraints of the pre-processing approach such as occlusion, appearance, illumination condition, and color intensity, among others.
- Finally, there’s also the event/behavior recognition process with both an object-based and holistic-based approach, both of which analyzes regular motion patterns from speed to direction, to not just analyze normal crowd behaviors but to also identify abnormal or anomalous crowd behaviors.
Bottom line
An in-depth understanding of crowd analysis and its applications is necessary to understand the value of computer vision applications. This is also necessary to answer the question of ‘how does computer vision work.’ Computer vision, as well as other technologies such as Wi-Fi counting, and thermal imaging, among others are engaged in people counting devices and crowd analysis techniques. Crowd analysis is also gaining more popularity by the day and has found prominent use cases in;
- Monitoring marketing effectiveness and conversion rates in retail stores.
- Analyzing traffic flows and patterns for crowd management in mass gatherings like stadiums and concerts.
- Monitoring high-traffic areas in shopping malls and stores.
- Analyzing visitor counts in museums, libraries, and similar establishments to make well-informed decisions on seasonal staffing and other similar strategic decisions, etc.
- Estimating the number of people inside a building for better fire management or fire response during outbreaks, etc
While the global market for crowd analytics is still in its early development phase, analysts predict an impressive growth with a CAGR of 25.8% that could increase revenues in this sector to as much as $2.4 billion by 2024.